While most people expect Quantum computers to be the ones that disrupt our conventional hardware, there’s still a lot of work to be done before they go mainstream. Neuromorphic computers, on the other hand, have a very real chance of upgrading us from our binary system of 1s and 0s.
The main difference between ChatGPT 3 and ChatGPT 4 is the scale (or number of parameters). In a recent JRE podcast, Jeremie and Edouard Harris, co-founders of Gladstone AI, talk about how every major player is throwing computational resources at its models in order to scale them up. They also talk about how dollars amount to IQ points when you’re scaling up these language models to make them understand language better. For example, while ChatGPT 3.5 was trained using 175 billion parameters, ChatGPT 4 was trained using over a trillion parameters and that’s why it’s so much better. Now the problem with throwing raw, conventional processing power at these neural networks is that it’s expensive and probably unsustainable. It costs millions of dollars a day to run ChatGPT, which is probably the reason why there is now a 20$ subscription involved.
Modeled after the human brain
According to a recent post on Forbes, deploying ChatGPT into every Google search would require close to half a million GPUs and would cost Google over $100 billion in capital expenses. Another recent study by the London-based International Electrotechnical Commission states that by 2027, the AI industry alone will consume more natural resources and electricity than a country the size of the Netherlands. That’s a lot of power for something that isn’t even up to human intelligence yet. Speaking of human intelligence, it is estimated that we possess in our heads an organ with a processing power of 1 exaflop with about 2.5 million gigs of RAM. Additionally, we use a millionth of the power consumed by supercomputers with similar specifications.
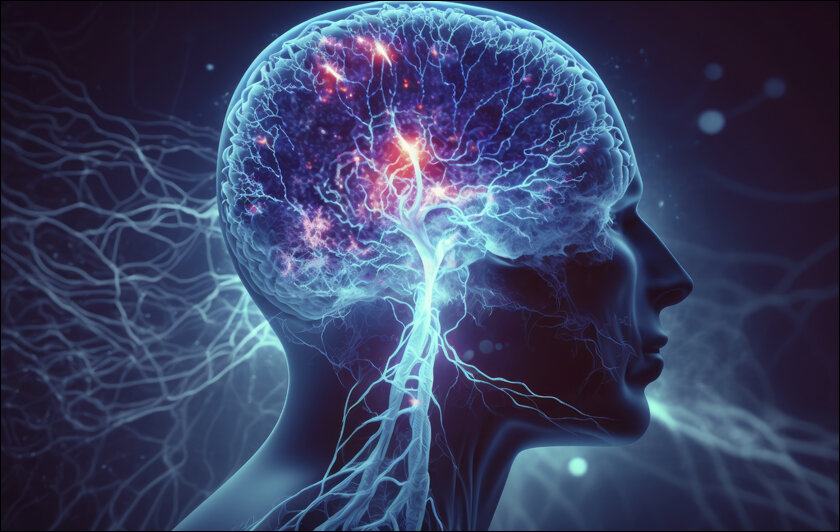
To quote Gordon Wilson, former CEO of Rain AI, “Our brain is “a million times” more efficient than the AI technology (hardware) that ChatGPT and large language models use.” This is probably what has scientists looking to the human brain for inspiration. No, we’re not talking about the neural networks that power the large language models like ChatGPT that we are becoming all too familiar with. Neural networks are software solutions that despite being modeled after the human brain, still run on conventional computers. Neuromorphic computers, on the other hand, are actual hardware versions of neural networks and are different from conventional computers in almost every way, including how they are built and how they operate.
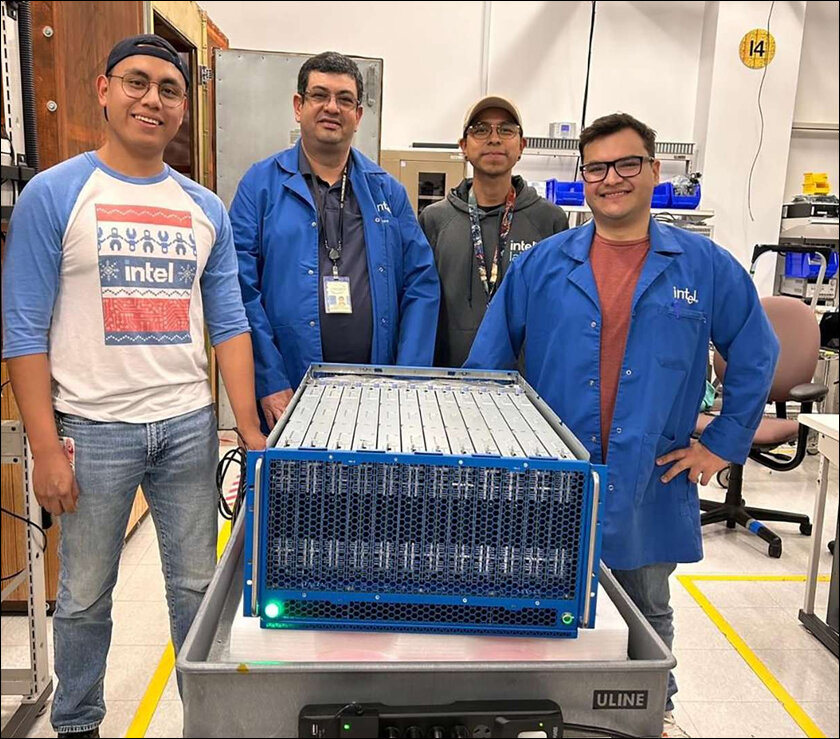
(Image Credit: Intel Corporation)
Intel’s Hala Point
While traditional computers are digital and use either 1s or 0s to represent data, neuromorphic computers can represent data using analog circuitry where an electric signal variation is used to mimic brain functions. Additionally, as opposed to traditional computers that have separate CPUs and RAM (and the limitations and bottlenecks that come along with that setup), neuromorphic computers have artificial neurons that can store and compute simultaneously (like the human brain). This not only eliminates bottlenecks but also vastly increases efficiency as they operate at extremely low voltages. A few months ago, Intel announced it had built Hala Point, the world’s largest neuromorphic computer that runs on Intel’s second-generation neuromorphic processors called Loihi 2 and is the size of a microwave oven.
Hala Point is the first large-scale neuromorphic computer that can run mainstream AI workloads and is built with 1152 Loihi chips which form over a billion artificial neurons with 128 billion synapses and over 140,000 processing cores. For reference, owls and vultures have roughly a billion neurons while we have about 86 billion. Intel claims that while working at full capacity, Hala Point is 20 times faster than a human brain, and up to 200 times faster when working at lower capacities. When processing mainstream AI workloads, Hala Point is 50 times faster than conventional CPU/GPU setups and uses 100 times less power. The overall efficiency translates to approximately 15 trillion 8-bit operations per second per watt.
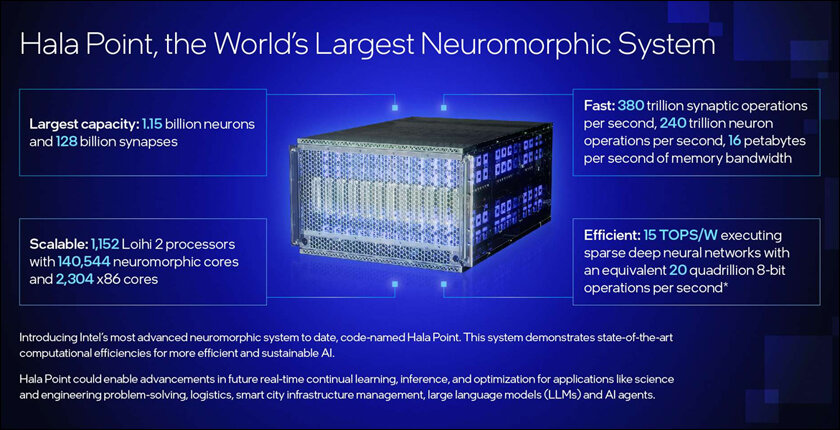
The future of hardware
While most people expect Quantum computers to be the ones that disrupt our conventional hardware, there’s still a lot of work to be done before they go mainstream. Neuromorphic computers, on the other hand, have a very real chance of upgrading us from our binary systems of 1s and 0s. Though Intel’s Hala Point is a research prototype and cannot be bought yet, Intel has granted access to over 200 Intel Neuromorphic Research Community (INRC) members that include educational institutions and government labs. There are also a number of neuromorphic systems in the works, a list of which can be viewed here. In conclusion, Neuromorphic computers represent a giant leap forward in computing capabilities while also a providing us with a realistic scenario in which we can sustainably develop AI.
In case you missed:
- This computer uses human brain cells and runs on Dopamine!
- Nvidia Project GROOT for humanoid robots
- Scientists gave a mushroom robotic legs and the results may frighten you
- Could the Future of Communication Be Holographic?
- AI in the Field of Scientific Discovery, Are We Ready?
- Could Quantum Gaming Become a Thing?
- How AI Is Helping Restore the World’s Coral Reefs
- These AI powered devices add smells to virtual worlds
- This Device can Actually Record Your Dreams Like a Movie!
- Introducing Nvidia’s L4 GPU profiles in the cloud