When Meta’s Chief AI scientist Yann LeCun was in India in October, he highlighted some interesting ideas about training AI and ML. Satyen K. Bordoloi delves into those and outlines how they could be game changers.
Yann LeCun, VP and Chief AI Scientist at Meta – owner of Facebook, Instagram, and WhatsApp – was in India in the last week of October, giving lectures and holding interactions. He proposed some unique ideas. On October 22, delivering the “Prof. Subra Suresh Institute Lecture Series” at IIT Madras, Chennai, he said: “If you are interested in human-level AI, don’t work on LLMs. Abandon generative models if you are in favour of human-level intelligence. Abandon probabilistic models in favour of energy-based models.” He was speaking on: ‘How Could Machines Reach Human-Level Intelligence?’.
Though AI has made remarkable strides with LLMs (Large Language Models) like ChatGPT and Gemini, and generative models based on GANs (Generative Adversarial Networks) and VAEs (Variational Autoencoders) leading the charge, there’s a growing sentiment among some AI researchers that the current trajectory may not be the most effective path towards achieving human-level AI. This perspective advocates a shift from certain popular approaches to more fundamental changes in AI research. What LeCun said fits right into that.
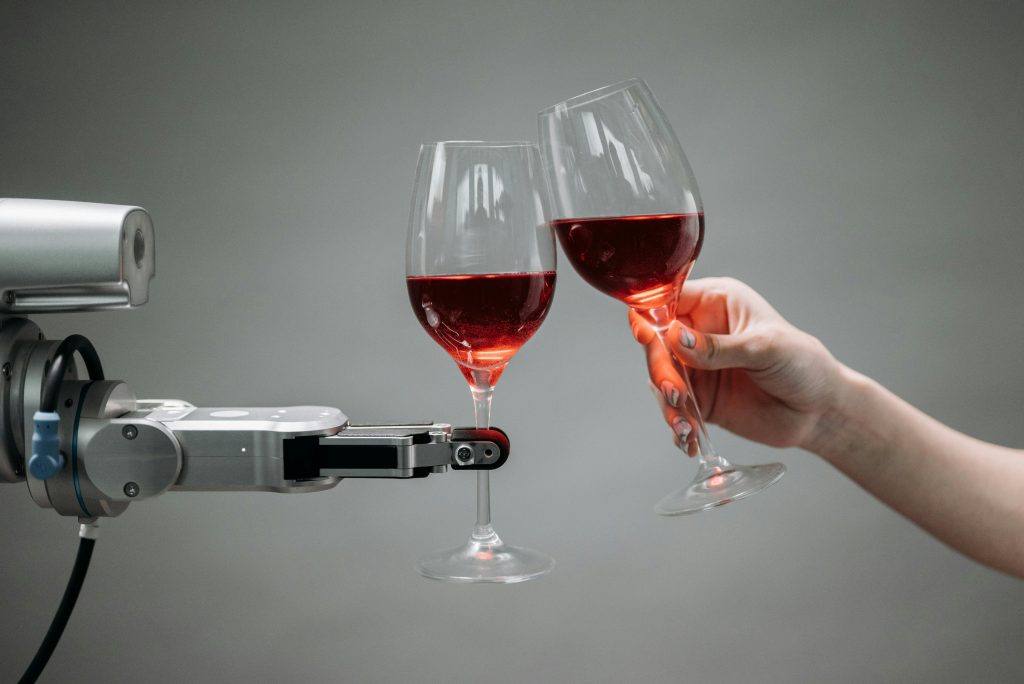
Moving Beyond LLMs: LLMs can process and generate human language, performing tasks such as explaining, translating, summarizing, composing music, and synthesizing images, which seem magical. However, LeCun, among other researchers, argues that LLMs may not be the most efficient route to achieving human-level intelligence. Generative models, including GANs and VAEs, generate new data by understanding and predicting existing patterns. While their core strength lies in exceptional pattern recognition and generating human-like text from those patterns, they lack the deeper understanding and reasoning capabilities common to human cognition.
LeCun says that to create AI that can think and reason like humans, focusing solely on LLMs might be limiting. LLMs are fundamentally designed to predict and generate sequences of text based on statistical patterns. They prioritize creativity and novelty over understanding and reasoning. While generative models can produce impressive outputs, they often do so without comprehending the underlying concepts. This limitation hinders their ability to perform tasks that require deep understanding and logical reasoning. To develop AI exhibiting human-like intelligence, researchers need to shift their focus from generative models to approaches emphasising cognitive abilities in the quest for better machine intelligence.
From Probabilistic to Energy-Based Models:
Probabilistic models have long been a cornerstone in AI development, relying on probability distributions to handle uncertainty and make predictions. These models excel in various applications, from speech recognition to machine translation. However, some researchers, including Yann LeCun, argue that they may not capture the full complexity of human cognition.
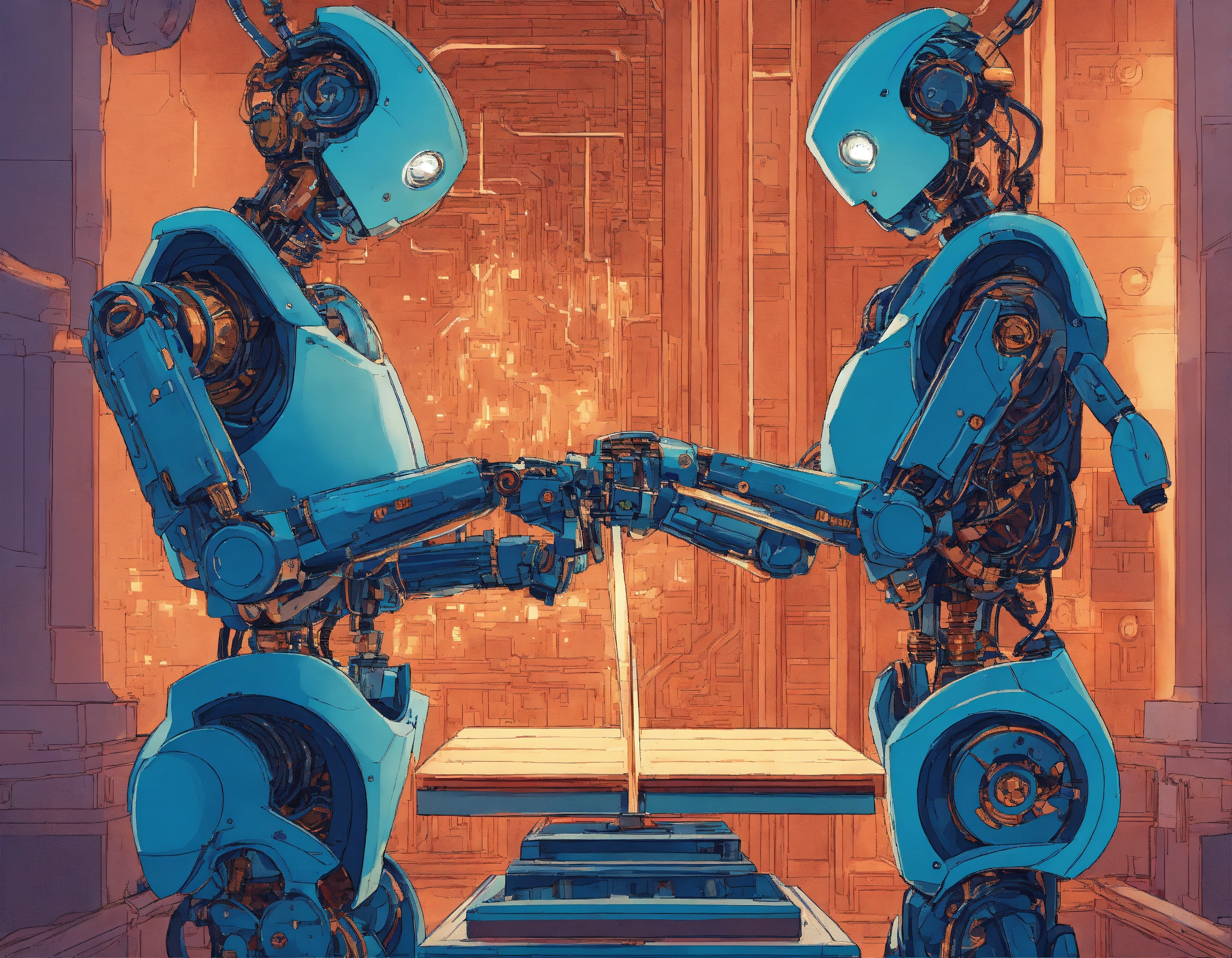
Energy-based models offer an alternative, focusing on assigning energy values to different data configurations, with lower energy states representing more likely scenarios. Inspired by statistical mechanics, this approach allows for modelling complex systems with many interacting components. The primary advantage of energy-based models is their ability to capture intricate relationships between variables without relying on explicit probabilistic reasoning.
Another key benefit is their flexible and robust learning from data. Unlike probabilistic models that depend on predefined distributions, energy-based models adapt to the underlying data structure. This adaptability is crucial for achieving human-level intelligence, as it aligns AI’s learning and reasoning processes more closely with human cognition.
Other Approaches:
Other AI researchers and developers are exploring various innovative approaches to achieve human-level reasoning. Take OpenAI’s new GPT model called o1, which integrates deep reasoning and thoughtful analysis directly into AI. Unlike traditional models that rely on pattern recognition, o1 uses chain-of-thought reasoning to break down complex problems into manageable chunks and solve them step-by-step. This approach allows the model to think like humans do, making it capable of tackling multistep problems with greater accuracy and depth than previous GPT models.
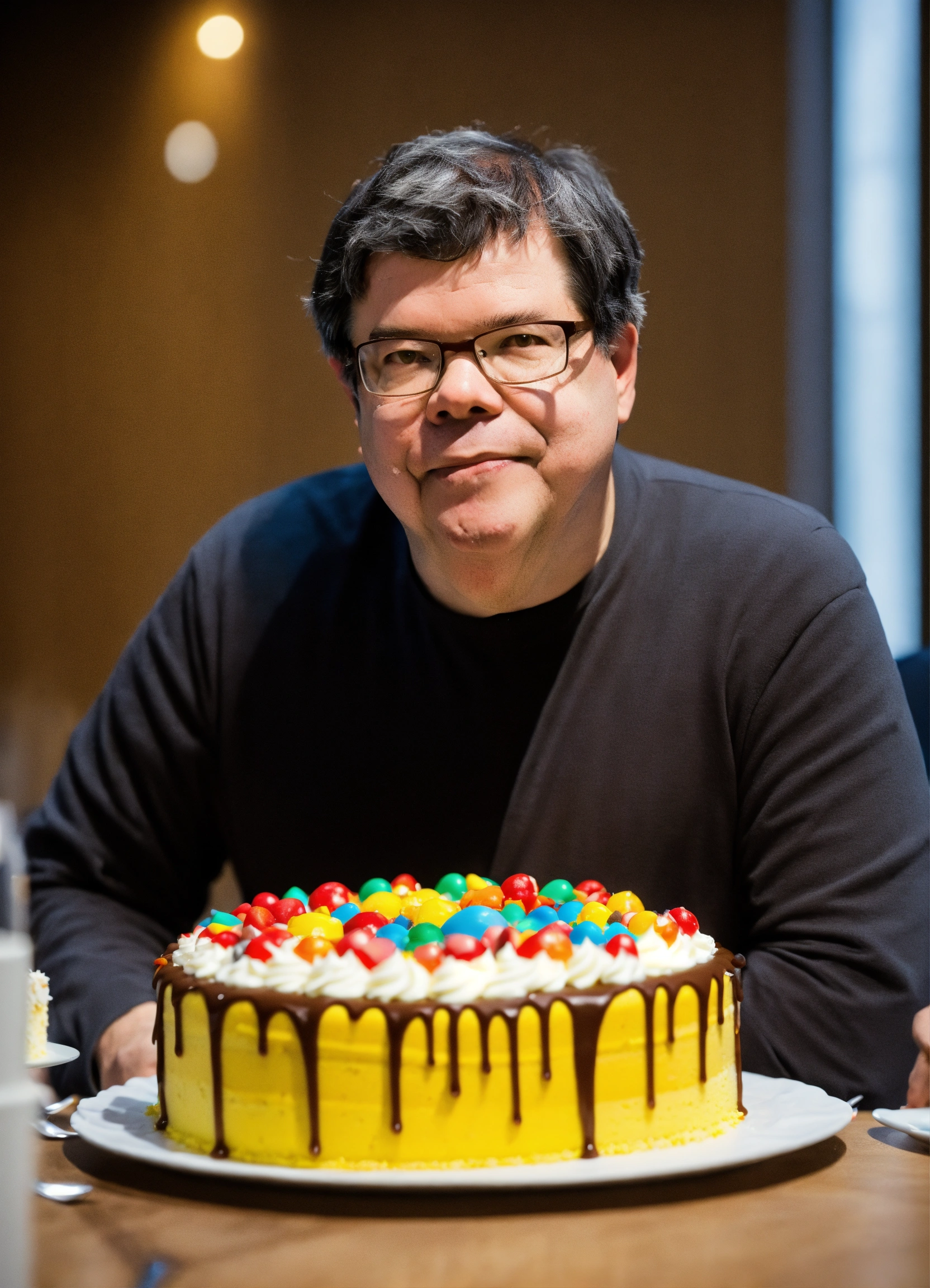
Additionally, some researchers are focusing on cognitive models and logic-based systems taken from other sciences to enhance the reasoning capabilities of AI. By embedding knowledge and logical reasoning into AI models instead of probabilistic approaches, they hope to develop systems that can autonomously execute multistep processes and make decisions based on a deeper understanding of the underlying principles.
The Path Forward:
Shifting AI research towards energy-based models reflects a belief that current methods, though effective, may not lead directly to human-level intelligence. Moving away from LLMs, generative models, and probabilistic models, allows researchers to explore alternative approaches that focus on understanding, reasoning, and embodiment. This does not negate the value of existing models, which remain crucial in various applications. However, to achieve human-level AI, the research community must remain open to new methodologies and frameworks.
The breakthrough for new models and methodologies will come from a collaborative effort not just across AI research communities, but interdisciplinary collaboration that combines insights from fields like neuroscience, cognitive science, and robotics. Open dialogue and knowledge sharing are essential to address the limitations and challenges of current models. Conferences, workshops, and collaborative research projects will play a key role in advancing the field.
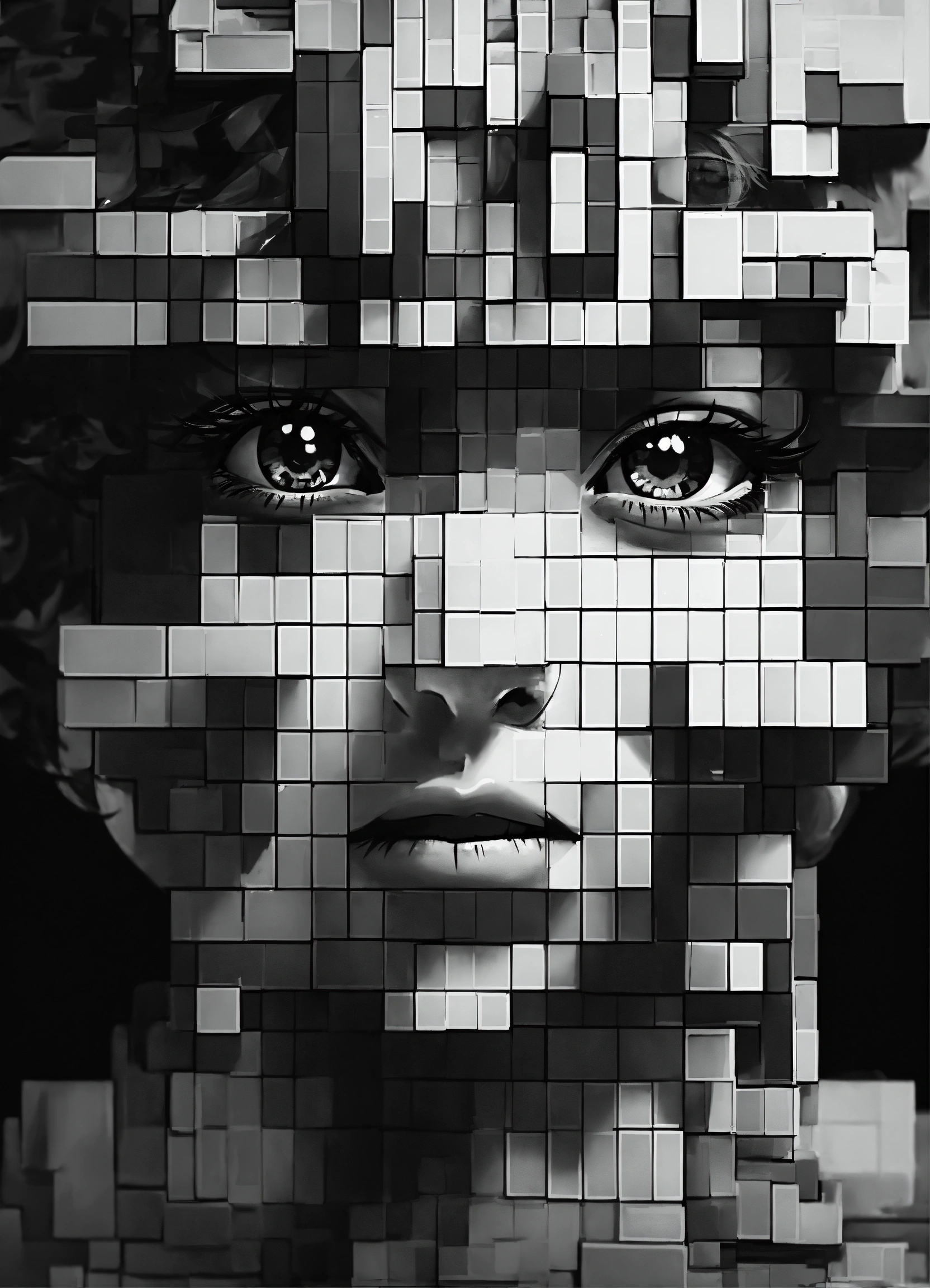
Ethical Considerations:
As researchers explore new approaches to AI, it is crucial to learn from the past. The current phase of AI development, around 12 years old – or seven if we consider the development of transformers and generative models – has raised considerable ethical concerns about how these models have been developed, trained, and used by companies. Ensuring that new AI systems are developed and deployed with respect for privacy, fairness, and transparency should be paramount. While AI development is crucial, establishing guidelines and best practices for its ethical development, training, and use is essential.
Though I have criticized doomsday scenarios proposed by some AI developers, it’s evident that researchers must be mindful of the potential societal impacts of human-level AI. Developing AI systems that can think and reason like humans raises complex issues, from the future of work to the dynamics of human-AI interactions and the potential for misuse. Addressing these concerns proactively is essential to ensure that AI technologies benefit society as a whole.
No one faces these ethical dilemmas more directly than Yann LeCun himself. While he may have good intentions, he works for a company and a boss – Mark Zuckerberg – known for handling technology-related issues unethically. For LeCun, and other researchers developing human-level reasoning, it seems charity must begin at home.
In case you missed:
- Pressure Paradox: How Punishing AI Makes Better LLMs
- OpenAI’s Secret Project Strawberry Points to Last AI Hurdle: Reasoning
- You’ll Never Guess What’s Inside NVIDIA’s Latest AI Breakthrough
- The Path to AGI is Through AMIs Connected by APIs
- How Does AI Think? Or Does It? New Research Finds Shocking Answers
- A Manhattan Project for AI? Here’s Why That’s Missing the Point
- Copy Of A Copy: Content Generated By AI, Threat To AI Itself
- The Great Data Famine: How AI Ate the Internet (And What’s Next)
- AI Hallucinations Are a Lie; Here’s What Really Happens Inside ChatGPT
- Bots to Robots: Google’s Quest to Give AI a Body (and Maybe a Sense of Humour)