That barely any content we consume is original, used to be a metaphysical and artistic angst, but with GenAI content in the mix for 2 years, researchers warn of a threat to AI itself, writes Satyen K. Bordoloi.
Few lines in literature have generated as much debate about their meaning as writer Chuck Palahniuk’s in Fight Club: ‘…everything is a copy of a copy of a copy.’ The protagonist says it, as he walks through life as a raging insomniac, unable to focus. The Hollywood film made the line and the book’s philosophy famous, but I don’t think anyone has yet connected that line to the modern world, especially the one created after GAI – Generative AI.
Today, most things we see and read are ‘a copy of a copy of a copy’, the reels of your friendly neighbourhood influencer included. This is fine, to a degree, as far as humans are concerned. But train an AI on content generated by AI systems, and you’ll see what is known as ‘model collapse’. This has been the finding of a research paper titled “AI models collapse when trained on recursively generated data” which explored what happens to AI systems trained only on AI content. The six researchers were from the University of Oxford, the University of Cambridge, Imperial College London, and the University of Toronto.
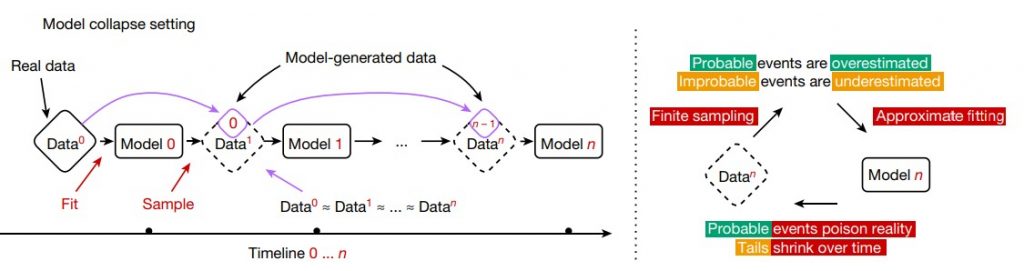
WHAT IS MODEL COLLAPSE
To put it simply, model collapse for AI systems is akin to dementia in humans, though the causes are different. Like human cognitive decline, AI systems trained on bad data will create bad data themselves. This polluted training set causes the subsequent system to misperceive reality. Thus, the results they’ll produce will not be occasional hallucinations, as most AI systems are prone to, but the only thing consistent with such a system trained on generations of data created only by AI is hallucination, rendering outputs of such a model to become utter junk, much like the gibberish of a human losing their cognitive functions.
The study highlights that such collapse affects various types of generative models, including large language models (LLMs), variational autoencoders (VAEs), and Gaussian mixture models (GMMs). When models are trained on data that includes a significant amount of AI-generated content, they begin to forget the nuances of the original human-generated data. What’s worse is that the defects in systems caused by such training on AI-generated data are irreversible, i.e., they cannot recover their original performance.
WHY THIS IS A PROBLEM
More and more content on the internet is being proven to be AI-generated. Another research found that more than half of the web content in certain languages – 57% – especially in less commonly spoken languages, is machine-translated. This pollution, as we wrote about earlier in Sify, is not just a problem for humans, but turns out even for machines. Like human cognitive functions decline with misinformation, disinformation, and lies fed to them, so does that of AI systems.
Despite many claiming AI systems have cognition, the truth is the opposite: they only regurgitate what is fed to them in different ways and patterns that make them seem unique. Thus, feed them bad data and these AI systems not only regurgitate worse, but it also messes up their continuous training as that is what these deep learning models do. Thus, the integrity of the entire system is compromised, rendering them useless.
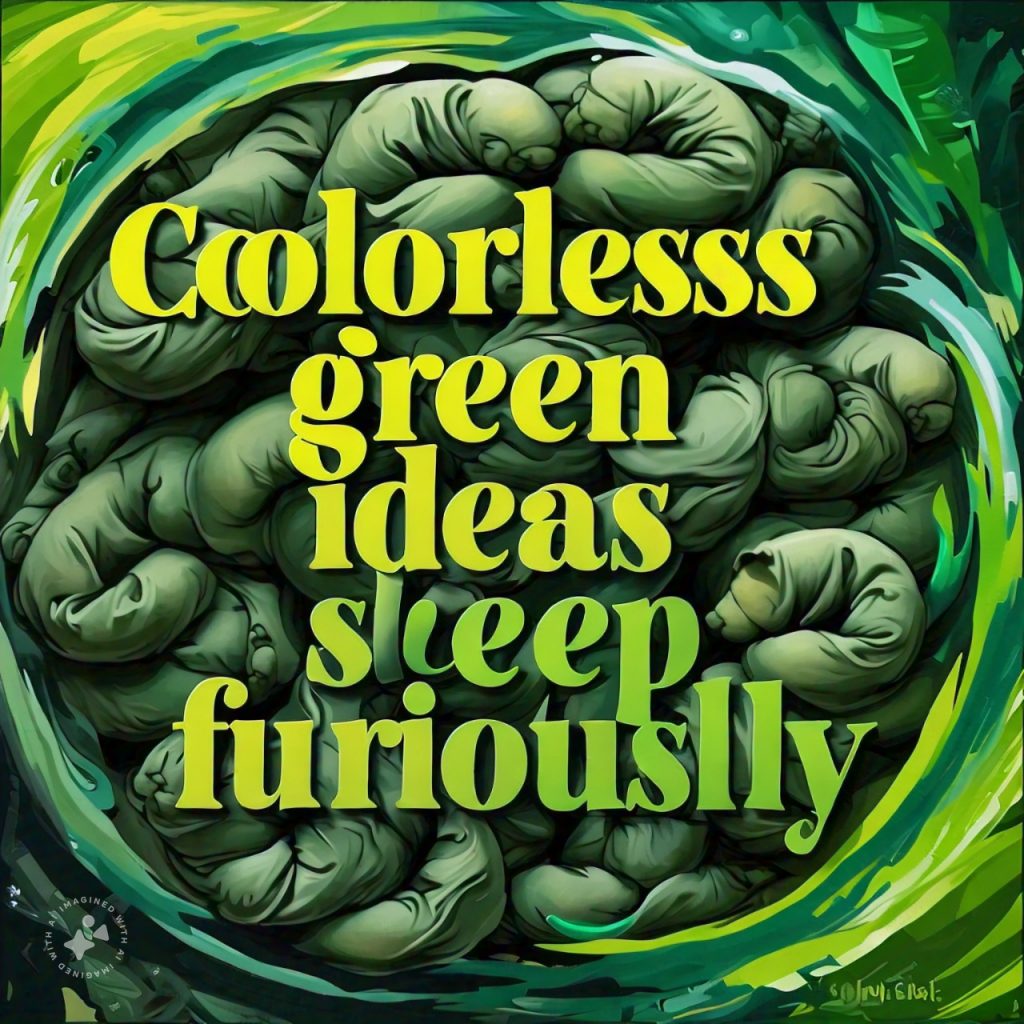
In 1957, linguist Noam Chomsky created a sentence that is grammatically correct but semantically nonsensical. The sentence was: “Colorless green ideas sleep furiously”. You can create your own, or Google them and discover a trove of sentences that are called ‘Garden-path sentences’. E.g. “The old man the boat.”, “The complex houses married and single soldiers and their families.”, “The prime number few.” etc. These are all grammatically correct, but meaningless and nonsensical. This is the sort of result you’ll get eventually from AI systems trained for generations on not human, but AI data.
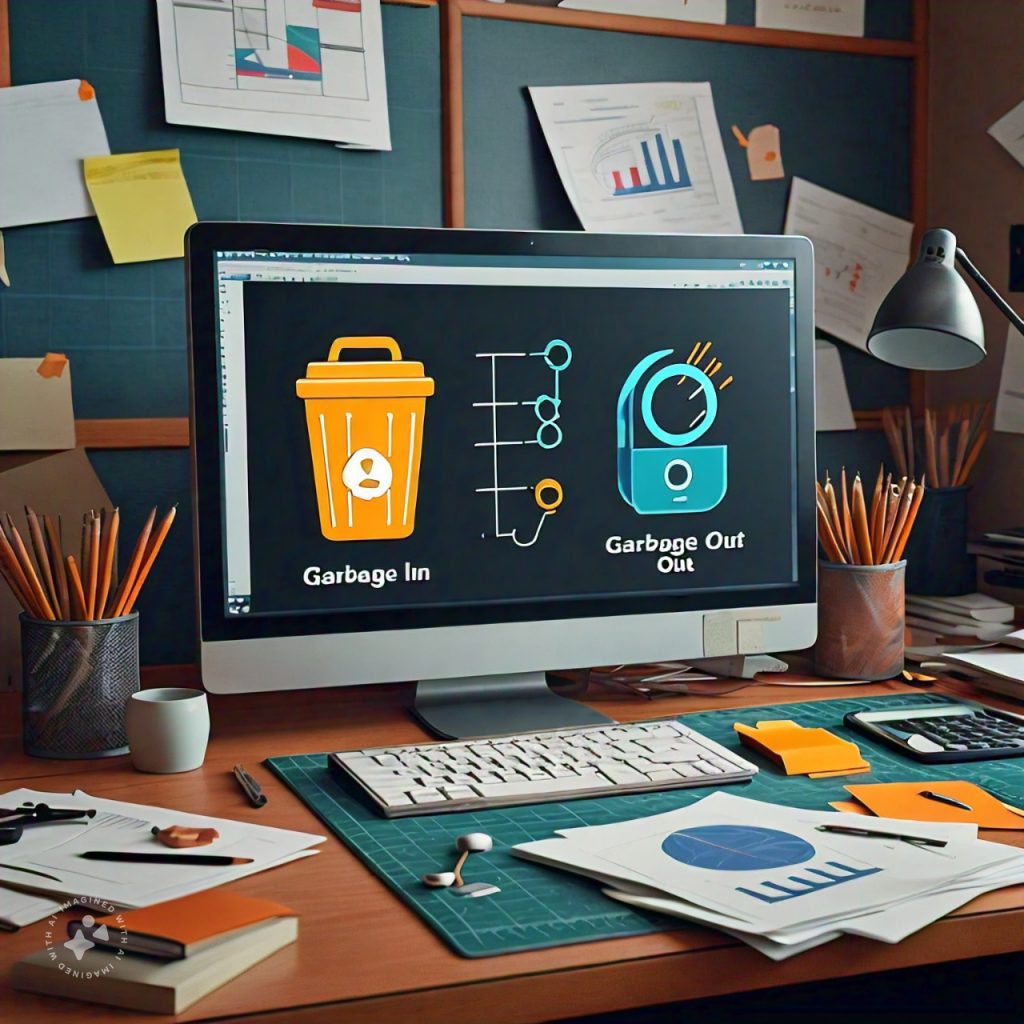
BIGGEST IRONY
That to me is the biggest irony of artificial intelligence systems: to be good enough for humans, the data AI is trained on has to come from humans. ‘AI see, AI do’ can be the mantra rehashed from ‘monkey see, monkey do’ to depict AI’s best ability: to mimic logic, intelligence, and meaning without having either of its own. There’s another term for this: Garbage In, Garbage Out (GIGO), one of the founding principles of computing i.e., if you feed a system garbage, the output will be the same – garbage. If one needed proof of the difference between humans and AI, this has to be it. Yet, I suspect, the gangs of AI doomsayers will only grow.
THE MOST PRICELESS COMMODITY IN THE AI WORLD
In the previous Sify article about content pollution in the modern world, I wrote that the data created before the age of generative AI is the only pure data we have. Thus, one of the most priceless commodities has already been scooped up by the early pioneers of Gen AI – internet content untouched by the pollution of generative AI. Data may be the new gold, but the purity of gold differs and the ones dug up before 2022, would be the most precious for AI developers as time passes.
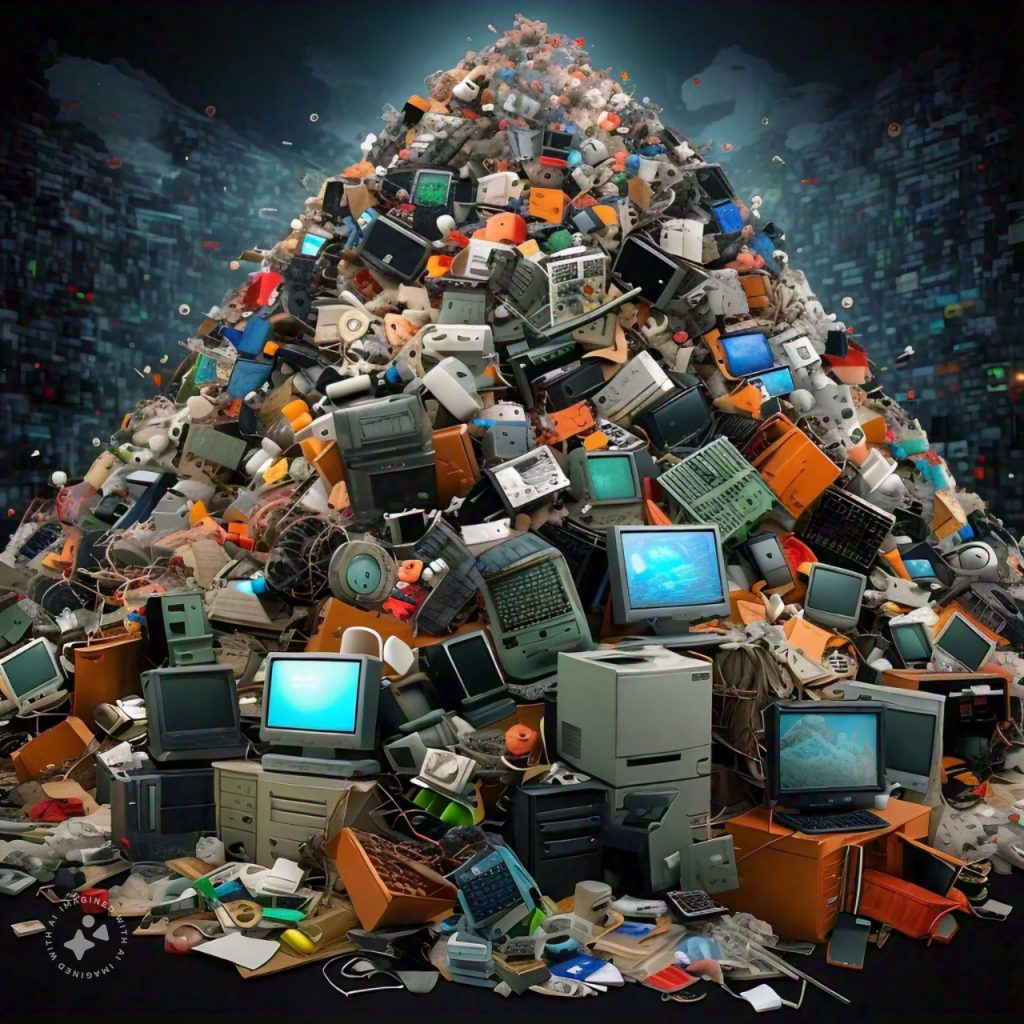
THREAT TO THE WORLD
Our world, more and more, relies on the ability of AI not only to do our repetitive tasks but also to dig out patterns that’ll solve a host of problems to save the world, from climate change to unique ways of deflecting asteroids. Then there are the AI pioneers talking about creating AGI – Artificial General Intelligence – AI systems that reach or exceed human intelligence. But how would they do that if the very data available to train AI systems are not good enough even to train generic AI systems? Though not a big problem right now, in the future it could be an existential one for humans.
The solution could be a new way not only of training AI models but also new ways of creating AI systems themselves. Progress has been made by different researchers, particularly by those at Google and Harvard who created a 3D nanoscale resolution map of a single cubic millimetre of the human brain. This 3D map of a pinprick of the human brain, which had over 57,000 cells, about 230 millimetres of blood vessels, and nearly 150 million synapses, generated 1.4 million GB of data. Maybe this sort of study of the human brain with an intent to mimic it in machines, i.e., neuromorphic computing, will help us create AI systems that model the human brain better to create better AI systems that won’t need so much data to train it.
The book ‘Fight Club’ was metaphoric in more ways than one. In it, the unnamed protagonist’s insomnia is triggered by the meaninglessness of modern life. Night after night as we lie swiping next on reels, our insomnia is precipitated by the meaninglessness of the content we consume, barely any of which is original. Everything is a copy of a copy of a copy. If ever there was a line to define the post-GAI world, this is it. What has been an utter surprise, though, is that this line would end up describing not just humans, but also AI. Fate, it seems, is not without a sense of wicked irony.
In case you missed:
- The Great Data Famine: How AI Ate the Internet (And What’s Next)
- Are Hallucinations Good For AI? Maybe Not, But They’re Great For Humans
- How Lionsgate-Runway Deal Will Transform Both Cinema & AI
- Rethinking AI Research: Shifting Focus Towards Human-Level Intelligence
- OpenAI’s Secret Project Strawberry Points to Last AI Hurdle: Reasoning
- AI vs. Metaverse & Crypto: Has AI hype lived up to expectations
- Quantum Leaps in Science: AI as the Assembly Line of Discovery
- The Path to AGI is Through AMIs Connected by APIs
- A Manhattan Project for AI? Here’s Why That’s Missing the Point
- AI Adoption is useless if person using it is dumb; productivity doubles for smart humans