January 2025 has had but one buzzword: DeepSeek. The brainchild of an eponymous Chinese AI lab, the DeepSeek AI assistant/model broke all records, rising to the top of multiple app store charts.
In fact, the much-spoken-about AI Assistant, powered by DeepSeek-V3, overtook its rival ChatGPT, becoming the top-rated free app on the iOS store in the United States. All this, along with the assertion that the LLM (large language model) cost just USD 5.6 million to train, has catapulted the AI model into the limelight – for the right and the not-so-right reasons. So, what is DeepSeek all about, how has it garnered international fame at such a lightning pace, and how is it threatening to upset the tech world order? Let’s delve into it.
DeepSeek’s Origins
DeepSeek is an AI development firm founded in May 2023 in Hangzhou, China, by Liang Wenfeng, who also co-founded High-Flyer, a Chinese quantitative hedge fund. Currently, DeepSeek is an independent AI research lab under High-Flyer’s umbrella, focusing on developing open-source LLMs. After the first model was released in November 2023, the company released multiple variations of its core LLM.
However, things turned around in January 2025 when the company released its R1 reasoning model, propelling the company into fame. The two features that caused the stir? Its cost-effectiveness and efficiency. The firm built its original open-source model by employing MoE (mixture-of-experts) training on other leading models.
Additionally, it supposedly trained its AI model without Nvidia’s high-end graphics chips essential for AI training (although it did use less-powerful Nvidia chips). This reportedly reduced its computational costs while keeping its performance at par with other leading LLMs for simple use cases. Plus, there are multiple services for its models, including API access, a mobile application, and a web interface.
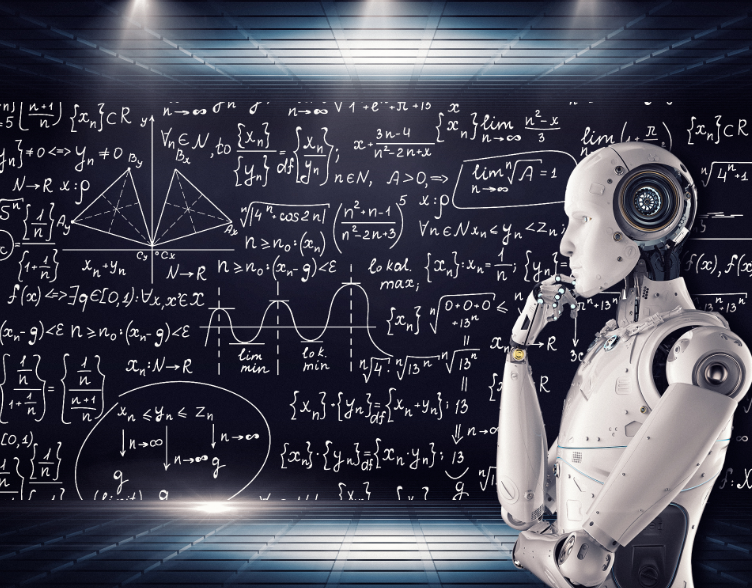
What Makes DeepSeek Tick – and Disruptive?
According to what DeepSeek claims, its main innovation is wielding its powerful, large models to use fewer resources and run just as well as other systems. The MoE system splits the larger model into submodels, each of which specializes in a specific data type or task. Accompanying this is a load-bearing system that dynamically shifts tasks from overworked to underworked submodels, as compared to other models that penalize already overburdened systems. It works with a dial within DeepSeek models called “inference-time compute scaling,” which amps up or down the allocated computing to match the complexity of assigned tasks.
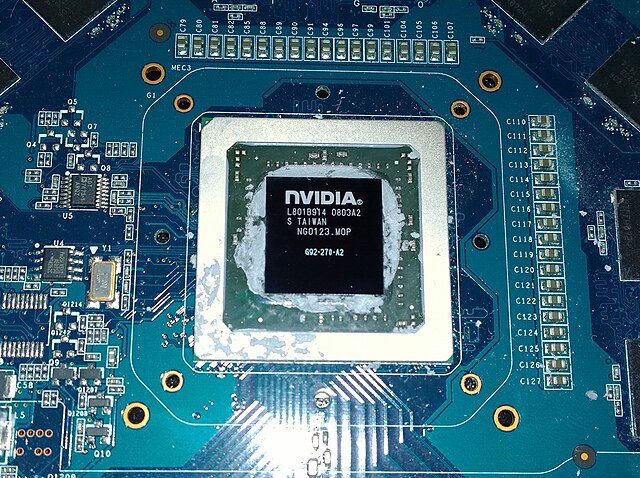
However, what changed the game was China’s access, or rather, limited access to Nvidia’s state-of-the-art H100 chips. So, according to DeepSeek, their models are designed using the “weaker” H800 chips, whose reduced chip-to-chip data transfer rate specially circumvents the export controls. It was the forced use of these less-powerful chips that resulted in DeepSeek’s mixed precision framework breakthrough, which allowed for faster training with fewer computational resources.
So, what does this startlingly efficient setup cost? According to company reps.
DeepSeek trained the current V3 in 2 months for – get this – a mere USD 5.58 million. This is peanuts compared to the tens of millions of dollars and several months that most of DeepSeek’s competitors have spent training their AI models. Similarly, V3’s running costs are also low — as much as 21 times cheaper to run than, let’s say Anthropic’s Claude, specifically Claude 3.5 Sonnet. DeepSeek’s advancements in reasoning capabilities are indicative of how significant the progress in AI development has been.
The Race Is On
The fact that DeepSeek could be built in less time, using less computation, and less monies, and could be run on less expensive machines locally, has had experts arguing that in the race towards bigger and better, they might have missed the opportunity to build smaller and smarter. V3 outperforms both – closed, via-API-only accessible models like OpenAI’s GPT-4o, and openly available and downloadable models like Meta’s Llama, according to DeepSeek’s internal benchmark testing.
DeepSeek’s R1 “reasoning” model is equally impressive, performing as well as OpenAI’s o1 model on key metrics, according to the company. Since it’s a reasoning model, R1 fact-checks itself, helping it avoid some significant pitfalls normally tripping up other AI models. While reasoning takes a little longer to arrive at solutions when compared to the usual non-reasoning models, they’re usually more reliable in arenas such as math, science, and physics.
Is DeepSeek Everything?
If DeepSeek does have a business model, what the model exactly is isn’t clear. The company prices its services and products well below the market value — and also gives others away for free. Additionally, there’s a unique downside to not just V3, but also R1 and other DeepSeek models. Since they’re AI developed by the Chinese, China’s internet regulator subjects them to internal benchmarking. The result? Its responses symbolize core socialist values. So, when you use DeepSeek’s chatbot app, let’s say R1 for instance, it won’t answer questions about Taiwan’s autonomy or Tiananmen Square.
That being said, the way DeepSeek is telling the tale, its efficiency breakthroughs have allowed the model to maintain its extreme cost competitiveness. While some experts caution that the figures supplied by the company are an underestimate, there’s no doubt about it that the implications are profound.
In case you missed:
- The Best Multi-Cloud Identity Management Practices
- Colocation Data Centres: An Overview
- Neural Networks vs AI – Decoding the Differences
- Can We Really Opt Out of Artificial Intelligence Online?
- Multiplexing in Networking: An Overview
- Six ways hosted private cloud adds value to enterprise business
- AI-Red Teaming: How Emulating Attacks Help Cybersecurity
- Why you should integrate disparate business systems: 5 key reasons
- Top cloud migration myths
- Everything you need to know about DaaS (Desktop as a Service)