Human genomes are complex, and artificial intelligence (AI) has stepped in to decode them further. Right from DNA sequencing to identifying genetic mutations, the rapid development of AI is significantly impacting the health industry and how we understand human genomes.
In the last few years, both interest in and funding for genomics and AI has grown, with the latter being touted as one of the critical technologies that can transform the healthcare industry. In fact, there are now businesses and industries relying on genomic data analysis for research and development of diagnostic decision-making and therapeutics. This article does a deep dive into the current trends in employing AI systems to extract useful data from highly complex genomic data and the challenges for the same.
Storing Bioinformatics Data
First and foremost, it is important to understand just how much data is being generated in genome sequencing. Roughly 100 GB of raw data is generated when a person’s whole genome is sequenced. Moreover, that more than doubles when the genome is sequenced using natural language processing and deep learning applications and algorithms. While the costs of sequencing human genomes continue to go down, the volumes of sequencing data are rising exponentially By 2025, it’s estimated that 40 EB (exabytes roughly equals 1,078 million GB) to store all human genome data. That is 8 times more storage required for storing every word spoken in history! The result? Genome analysis pipelines are deeply struggling to keep up with these massive data levels and methods to analyse them.
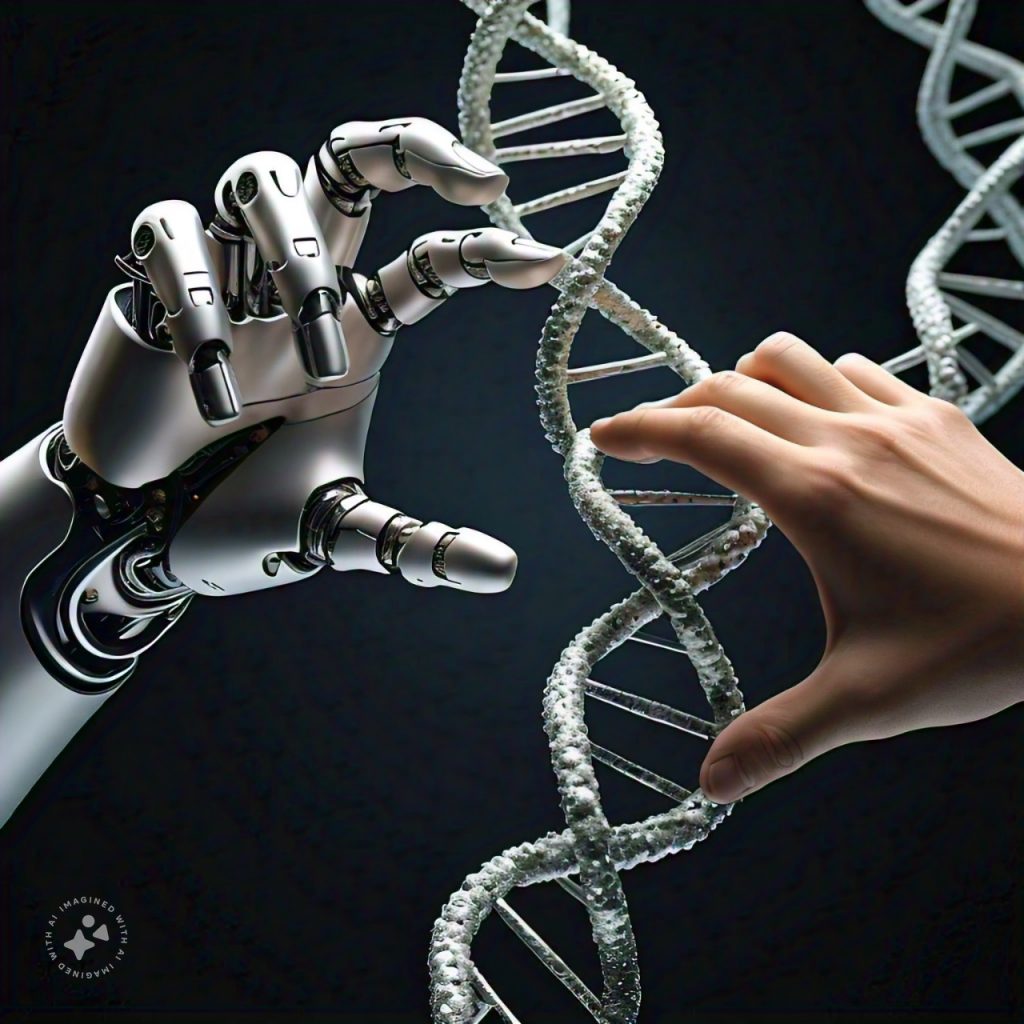
Harnessing the Power of AI for Analysing Genomics
The application of AI to the world of genomics, aptly dubbed AI-powered genomics, is and could have many useful applications in healthcare. AI algorithms such as deep learning (DL) and machine learning (ML) are used in genomic analysis for processing and interpreting large amounts of genetic data. They can not only identify patterns but also classify genetic variations and make predictions based on data extracted from large datasets.
Faster Genome Sequencing Analysis
Sequencing analysis of genomes is not just complicated but also a computationally intensive process. There are numerous steps that need to be performed to identify genetic variants in a human genome. AI models can quickly analyse epigenetic, gene expression, and genomic data to identify genetic mutations and their potential effects on the DNA.
What’s more, it is superior to traditional genome analysis methods in many ways, including ensuring biomarker discovery, personalized medicine, identification of novel mutations, efficient analysis, and improved accuracy. It also ensures that the entire process occurs closer to real-time, thus quickening the entire genomics workflow.
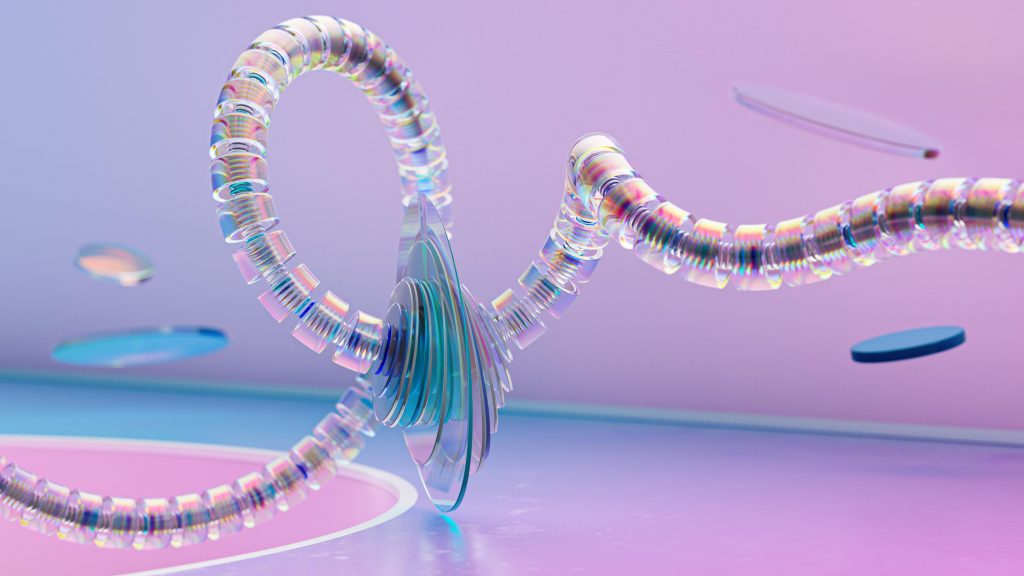
An artist’s illustration of artificial intelligence (AI). This image represents storage of collected data in AI. It was created by Wes Cockx as part of the Visualising AI project launched (Image Courtesy: Pexels.com)
Uncovering Genetic Variants
Variant calling is one of the most critical stages of genome sequencing projects. It is when researchers identify differences between a reference genome and a patient’s sample, thus helping clinicians determine what specific genetic disease a critically ill patient could possibly have.
Furthermore, it also helps researchers look through a population to discover and pinpoint newer drug targets. This helps further in personalised genetics, where the analysis of an individual’s genetic make-up could be used to ‘personalise’ their treatment plan. Or, it could also help in predicting the probability of certain people developing certain specific health conditions.
Assisting in genome-wide association studies
Phenotypes are a person’s observable traits, such as blood type, eye colour, and height, and are determined by both environmental factors and their genotype, or genomic makeup. Genome-wide association studies (GWAS) have identified millions of variants associated with these complex pathological phenotypes, and determining their functional effects has been a major challenge.
In this case, DL and ML algorithms have emerged as invaluable tools for addressing this challenge. They have been able to prioritized is ease-relevant and functional variants within different pathogenic contexts. Moreover, they can potentially predict the effects of previously unobserved or rare mutations as well. Finally, it could even analyse publicly available data from GWAS and prioritize causal variants associated with traits or diseases, which could be life-changing for people with immune-related diseases.
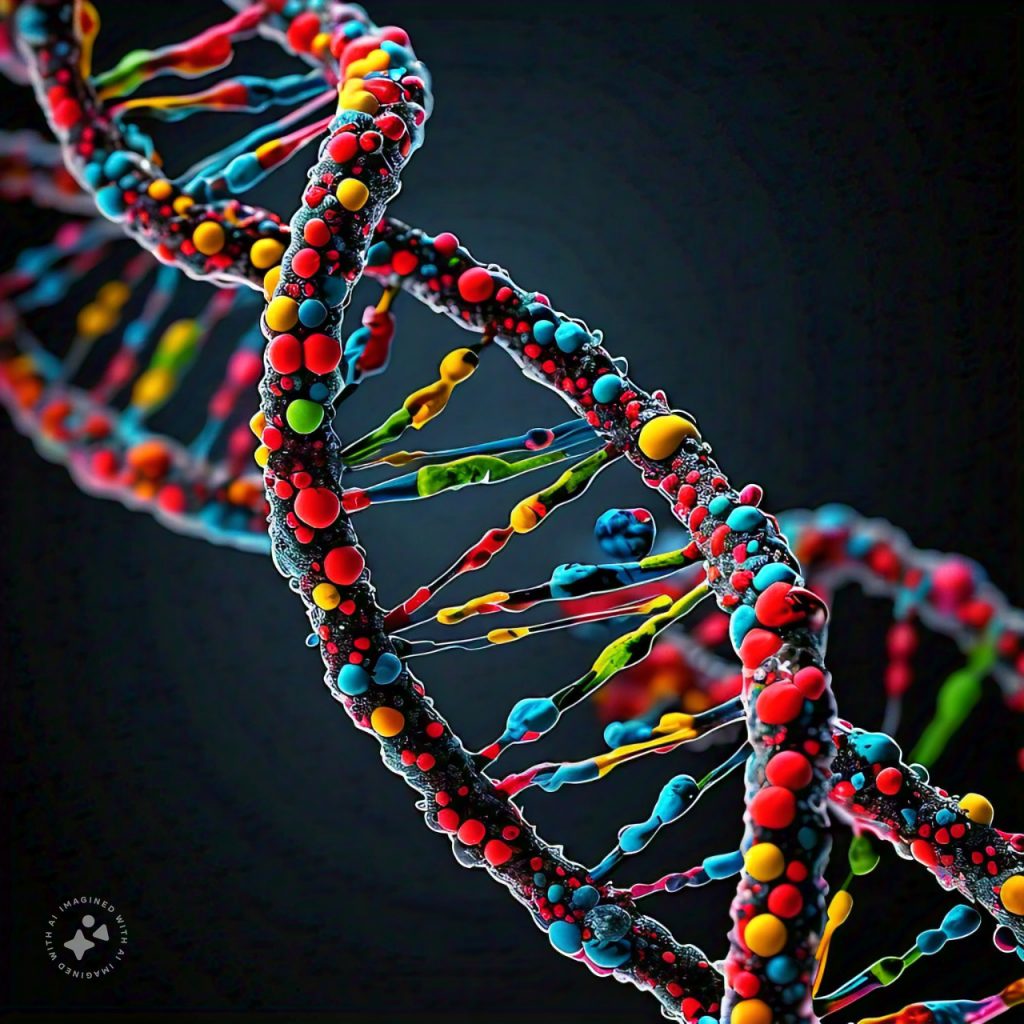
The future of genomics and AI
Advancements in genome sequencing have clearly ignited a revolution in digital biology.AI algorithms is now a huge part of preventive medicine, having demonstrated and continuing to demonstrate the ability to predict the impact of mutations on gene expression accurately. Future progress could even go as far as extending and enhancing these models to improve usefulness and accuracy in various genetic contexts, which could also mean designing new models.
AI is also increasingly playing a huge and crucial role in advancing personalized medicine by analysing genomic data to identify disease risks specific to individuals, as well as treatment responses and the best therapeutic approaches. It is showing its usefulness in creating precise and accurate models for forecasting disease development involving environmental factors and genetics.
Moreover, AI algorithms could also help researchers gain a comprehensive understanding of complex biological processes and uncover disease mechanisms, leading to the drug discovery and identification of therapeutic targets.
In case you missed:
- Neural Networks vs AI – Decoding the Differences
- AI Just Found a Lost City, is Archaeology Seeing a Digital Revolution?
- AI-Red Teaming: How Emulating Attacks Help Cybersecurity
- AI and Networking Infrastructure – 2024 Trends
- Wildlife Conservation: Is AI Changing It For The Better?
- AI Firewalls and How They Protect Your Data
- Can We Really Opt Out of Artificial Intelligence Online?
- IoT and its role in energy transition
- Top cloud migration myths
- The Best Multi-Cloud Identity Management Practices